May 24, 2024
EHA 2024 - AI for Enhanced Bone Marrow Analysis

Korsuk Sirinukunwattana
CTO
We're delighted to announce two of our abstracts have been accepted for presentation at the European Hematology Association (EHA) 2024 Conference.
Join us for the poster session on Friday, June 14 18:00–19:00 CEST
Xuezi Hu and Saad Bashir, from our machine learning team, have developed proof of concept AI-based methods to enhance the accuracy and safety of bone marrow assessments and predict CALR mutations from routine H&E stained bone marrow samples in myeloproliferative neoplasms (MPNs).
Enhancing the accuracy and safety of bone marrow assessments

Xuezi Hu
Machine Learning Scientist
In clinical practice, pathologists often report on tissue samples of varying quality; a subset of samples may not sufficiently meet the quality standards for clinical reporting. This can lead to unreliable diagnosis, impacting crucial clinical decisions.
Our study addresses the issue of quality in whole slide images by integrating rigorous quality control steps within our AI workflows, thus enhancing the precision and consistency of bone marrow trephine (BMT) examinations. The promising results also highlight the critical minimum tissue area for reliable BMT evaluations.
We thank our exceptional Ground Truth Labs team, advisors, and partners for their support and shared commitment to our mission.
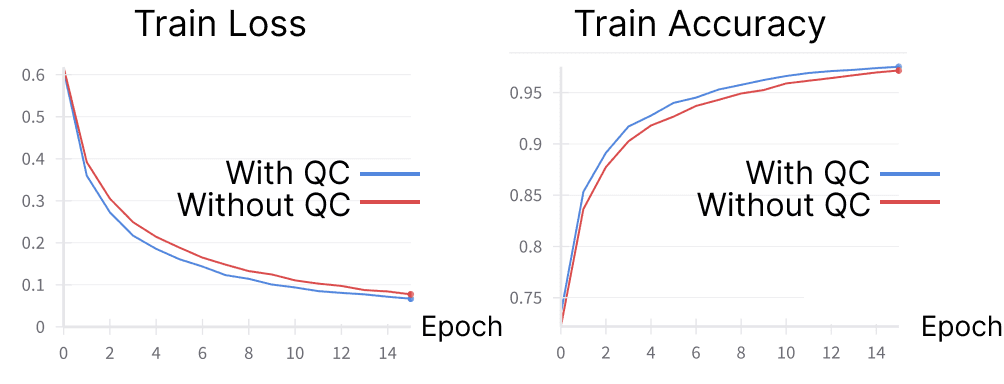
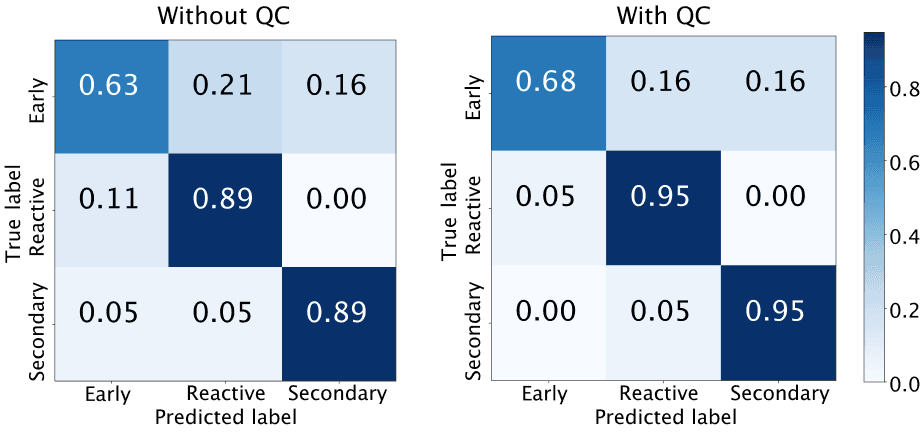
See the full abstract and learn more about the work on the EHA 2024 website.
Predicting CALR mutations from H&E bone marrow samples

Saad Bashir
Machine Learning Scientist
CALR mutations, associated with improved survival rates in Myeloproliferative Neoplasms (MPN, a rare group of blood cancers), have been a focal point in developing targeted therapies for MPN. These include innovative approaches like blocking antibodies, T-cell redirecting immune therapies, and novel vaccination strategies. Our work demonstrates the potential of predicting mutational status based on morphological changes observed in the tissue.
Critically, the model not only predicts CALR mutational status, it also identifies specific regions within the tissue that correlate strongly with this mutation. While it's known that CALR mutations lead to fibrosis and changes in megakaryocytes, the underlying mechanisms remain unknown. Our research opens new avenues for exploring these relationships through a morphological lens.
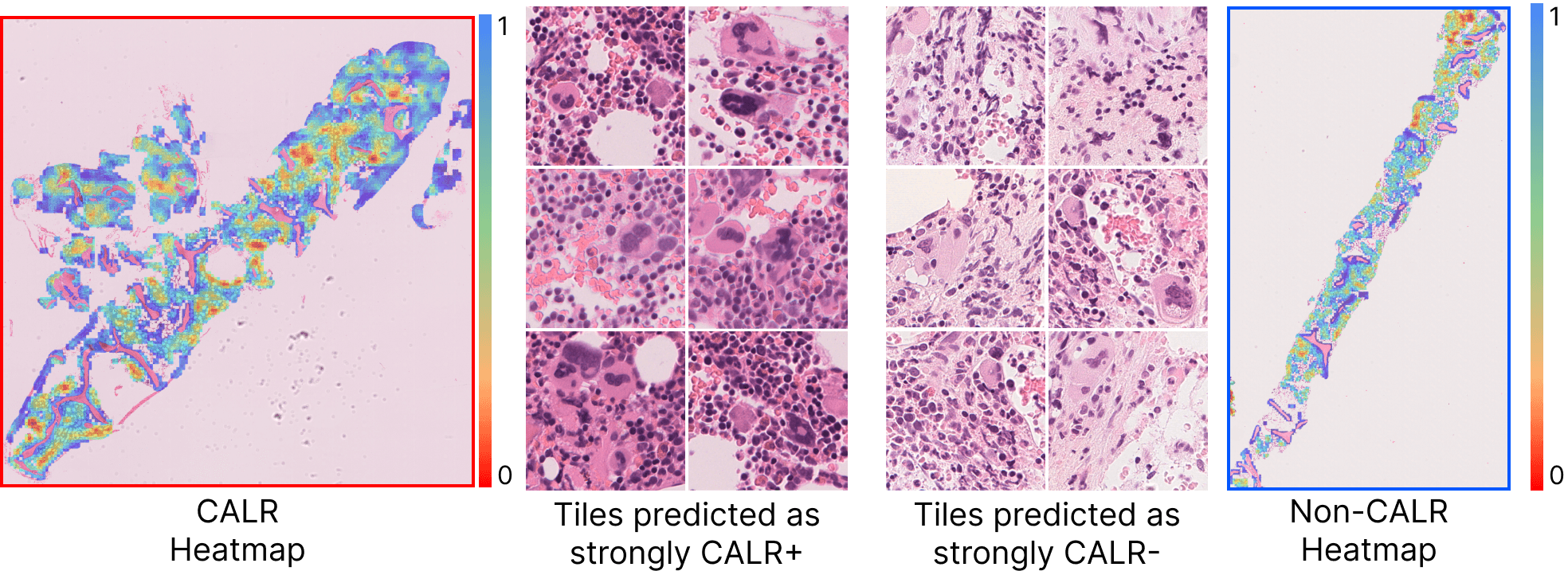
See the full abstract and learn more about the work on the EHA 2024 website.